CULane Dataset
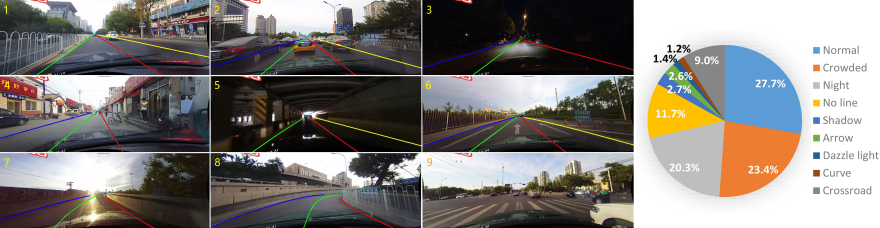
Description
CULane is a large scale challenging dataset for academic research on traffic lane detection. It is collected by cameras mounted on six different vehicles driven by different drivers in Beijing. More than 55 hours of videos were collected and 133,235 frames were extracted. Data examples are shown above. We have divided the dataset into 88880 for training set, 9675 for validation set, and 34680 for test set. The test set is divided into normal and 8 challenging categories, which correspond to the 9 examples above.
For each frame, we manually annotate the traffic lanes with cubic splines. For cases where lane markings are occluded by vehicles or are unseen, we still annotate the lanes according to the context, as shown in (2)(4). We also hope that algorithms could distinguish barriers on the road, like the one in (1). Thus the lanes on the other side of the barrier are not annotated. In this dataset we focus our attention on the detection of four lane markings, which are paid most attention to in real applications. Other lane markings are not annotated.
For more details, please refer to our paper.
Downloads
You can download the dataset from Google Drive or Baidu Cloud (If you use Baidu Cloud, make sure that images in driver_23_30frame_part1.tar.gz and driver_23_30frame_part2.tar.gz are located in one folder 'driver_23_30frame' instead of two seperate folders after you decompress them. This should naturally be the case if you decompress the two files with the default setting. ).
The dataset folder should include:
1. Training&validation images and annotations:
  - driver_23_30frame.tar.gz
  - driver_161_90frame.tar.gz
  - driver_182_30frame.tar.gz
For each image, there would be a .txt annotation file, in which each line gives the x,y coordinates for key points of a lane marking.
2. Testing images and annotations:
  - driver_37_30frame.tar.gz
  - driver_100_30frame.tar.gz
  - driver_193_90frame.tar.gz
3. Training/validation/testing list:
  - list.tar.gz
For train_gt.txt, which is used for training, the format for each line is "input image, per-pixel label, four 0/1 numbers which indicate the existance of four lane markings from left to right".
4. Lane segmentation labels for train&val:
  - laneseg_label_w16.tar.gz
  which are generated from original annotations.
Note: The raw annotations (not segmentation labels) for training&val set are not correct before April 16th 2018. To update to the right version, you could either download "annotations_new.tar.gz" and cover the original annotation files or download the training&val set again.
Tools
1. To evaluate your method, you may use evaluation code in this repo.
2. To generate per-pixel labels from raw annotation files, you could use this code.
Contact
Should you have any question about this dataset, please send email to xingangpan1994@gmail.com.
License
This dataset is made freely available to academic and non-academic entities for non-commercial purposes such as academic research, teaching, scientific publications, or personal experimentation. Permission is granted to use the data given that you agree:
1. That the dataset comes “AS IS”, without express or implied warranty. Although every effort has been made to ensure accuracy, we (SenseTime Group Limited) do not accept any responsibility for errors or omissions.
2. That you include a reference to the CULane Dataset in any work that makes use of the dataset.
3. That you do not distribute this dataset or modified versions. It is permissible to distribute derivative works in as far as they are abstract representations of this dataset (such as models trained on it or additional annotations that do not directly include any of our data) and do not allow to recover the dataset or something similar in character.
4. That you may not use the dataset or any derivative work for commercial purposes as, for example, licensing or selling the data, or using the data with a purpose to procure a commercial gain.
5. That all rights not expressly granted to you are reserved by us (SenseTime Group Limited).
Citation
@inproceedings{pan2018SCNN, author = {Xingang Pan, Jianping Shi, Ping Luo, Xiaogang Wang, and Xiaoou Tang}, title = {Spatial As Deep: Spatial CNN for Traffic Scene Understanding}, booktitle = {AAAI Conference on Artificial Intelligence (AAAI)}, month = {February}, year = {2018} }
Acknowledgments
Most work for building this dataset is done by Xiaohang Zhan, Jun Li, and Xudong Cao. We thank them for their contribution.