Exploiting Deep Generative Prior for Versatile Image Restoration and Manipulation
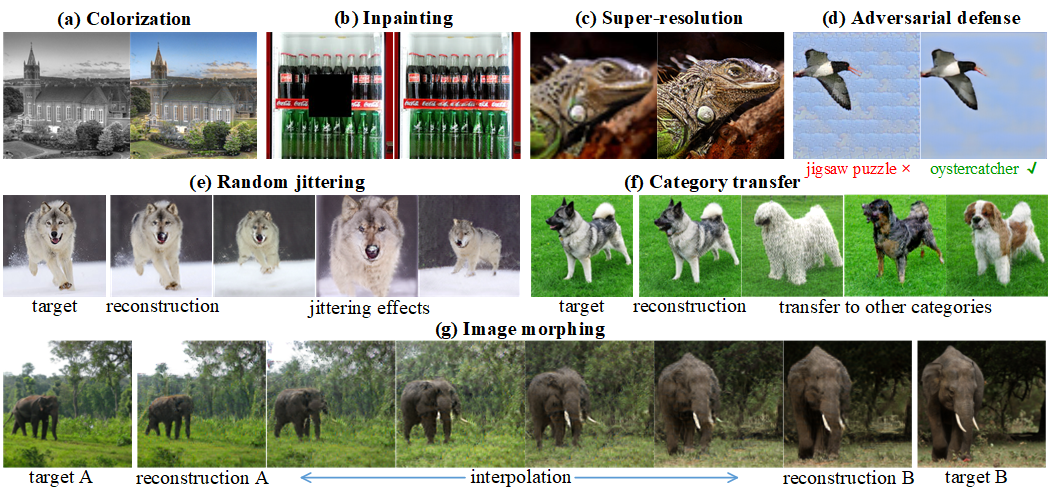
Abstract
Learning a good image prior is a long-term goal for image restoration and manipulation. While existing methods like deep image prior (DIP) capture low-level image statistics, there are still gaps toward an image prior that captures rich image semantics including color, spatial coherence, textures, and high-level concepts. This work presents an effective way to exploit the image prior captured by a generative adversarial network (GAN) trained on large-scale natural images. As shown in Fig.1, the deep generative prior (DGP) provides compelling results to restore missing semantics, e.g., color, patch, resolution, of various degraded images. It also enables diverse image manipulation including random jittering, image morphing, and category transfer. Such highly flexible restoration and manipulation are made possible through relaxing the assumption of existing GAN-inversion methods, which tend to fix the generator. Notably, we allow the generator to be fine-tuned on-the-fly in a progressive manner regularized by feature distance obtained by the discriminator in GAN. We show that these easy-to-implement and practical changes help preserve the reconstruction to remain in the manifold of nature image, and thus lead to more precise and faithful reconstruction for real images. Code is available at https://github.com/XingangPan/deep-generative-prior.
Video
Demos
Image restoration
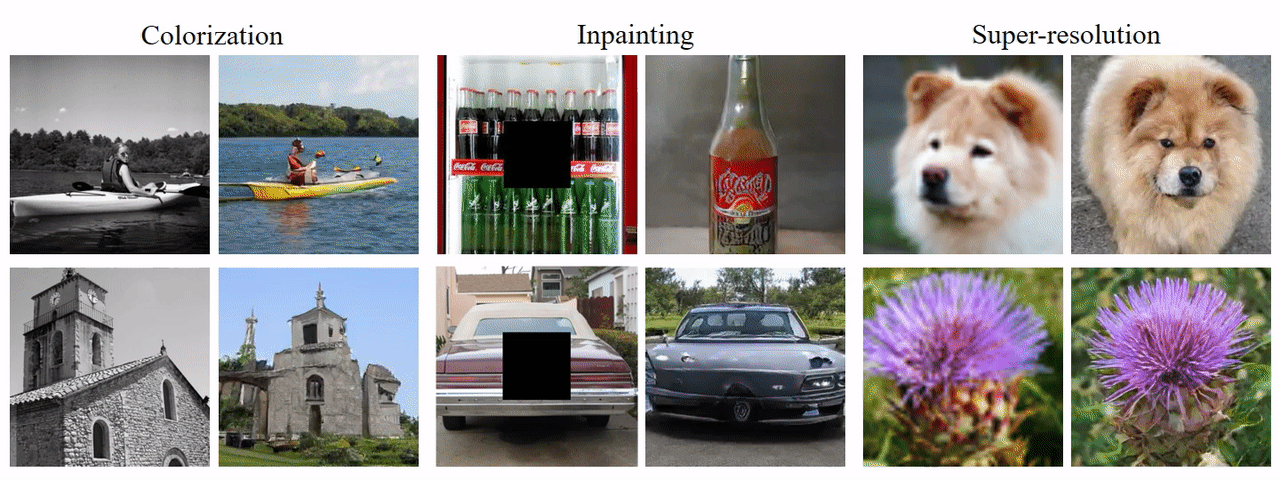
Image manipulation
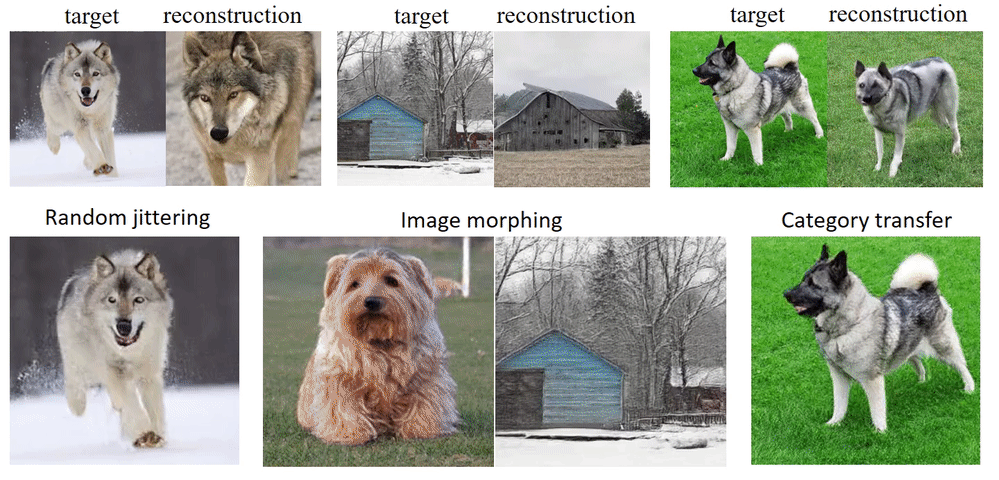
Materials
Code
Citation
@inproceedings{pan2020dgp, author = {Pan, Xingang and Zhan, Xiaohang and Dai, Bo and Lin, Dahua and Loy, Chen Change and Luo, Ping}, title = {Exploiting Deep Generative Prior for Versatile Image Restoration and Manipulation}, booktitle = {European Conference on Computer Vision (ECCV)}, year = {2020} }
@ARTICLE{pan2020dgp_pami, author={Pan, Xingang and Zhan, Xiaohang and Dai, Bo and Lin, Dahua and Loy, Chen Change and Luo, Ping}, journal={IEEE Transactions on Pattern Analysis and Machine Intelligence}, title={Exploiting Deep Generative Prior for Versatile Image Restoration and Manipulation}, year={2021}, volume={}, number={}, pages={1-1}, doi={10.1109/TPAMI.2021.3115428} }