Do 2D GANs Know 3D Shape? Unsupervised 3D Shape Reconstruction from 2D Image GANs
ICLR 2021 (Oral)
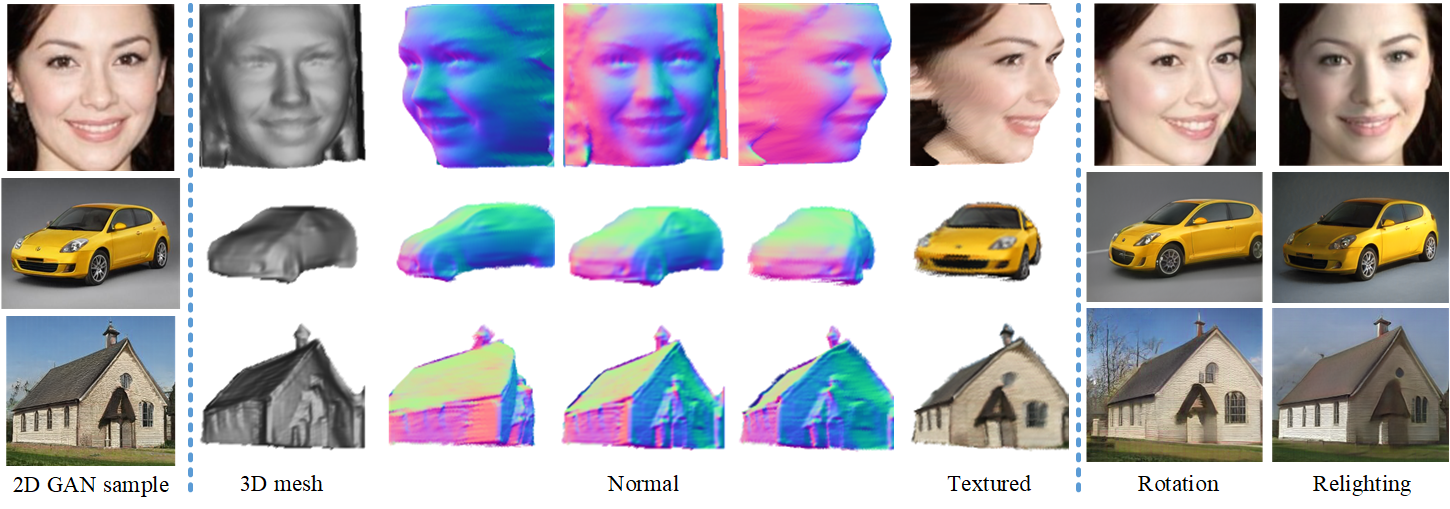
Abstract
Natural images are projections of 3D objects on a 2D image plane. While state-of-the-art 2D generative models like GANs show unprecedented quality in modeling the natural image manifold, it is unclear whether they implicitly capture the underlying 3D object structures. And if so, how could we exploit such knowledge to recover the 3D shapes of objects in the images? To answer these questions, in this work, we present the first attempt to directly mine 3D geometric clues from an off-the-shelf 2D GAN that is trained on RGB images only. Through our investigation, we found that such a pre-trained GAN indeed contains rich 3D knowledge and thus can be used to recover 3D shape from a single 2D image in an unsupervised manner. The core of our framework is an iterative strategy that explores and exploits diverse viewpoint and lighting variations in the GAN image manifold. The framework does not require 2D keypoint or 3D annotations, or strong assumptions on object shapes (e.g. shapes are symmetric), yet it successfully recovers 3D shapes with high precision for human faces, cats, cars, and buildings. The recovered 3D shapes immediately allow high-quality image editing like relighting and object rotation. We quantitatively demonstrate the effectiveness of our approach compared to previous methods in both 3D shape reconstruction and face rotation. Our code and models will be released at https://github.com/XingangPan/GAN2Shape.
Demo
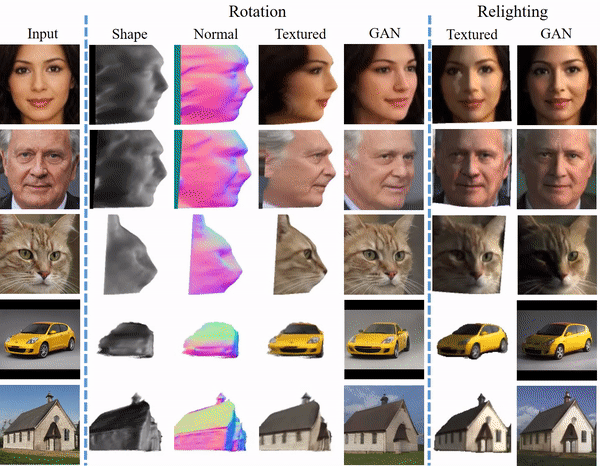
Recovered 3D shape and rotation&relighting effects using GAN2Shape.
Method Overview
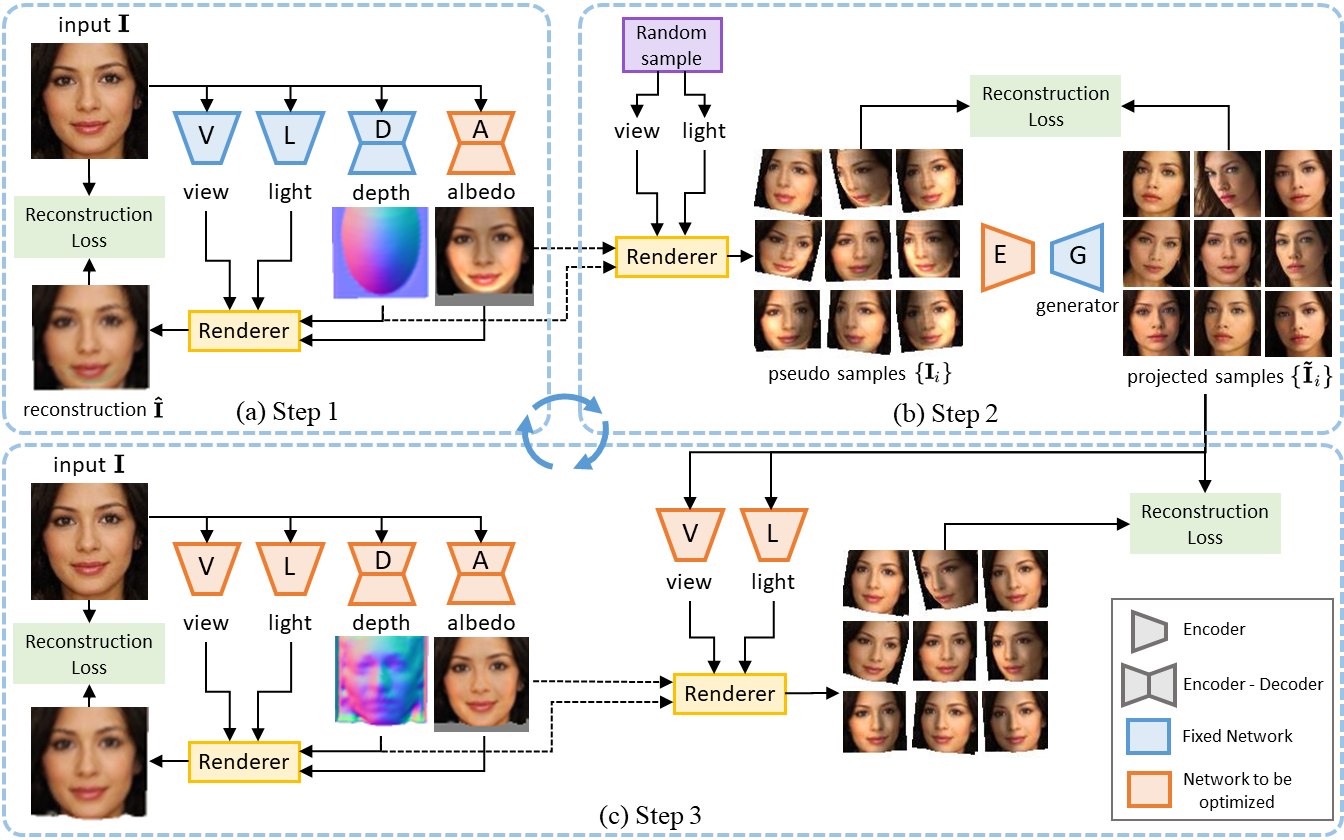
(a) Given a single image, Step 1 initializes the depth with ellipsoid, and optimizes the albedo network A. (b) Step 2 uses the depth and albedo to render `pseudo samples' with various random viewpoint and lighting conditions, and conducts GAN-inversion to them to obtain the `projected samples'. (c) Step 3 refines the depth map by optimizing (V, L, D, A) networks to reconstruct the projected samples. The refined depth and models are used as the new initialization to repeat the above steps.
More Results
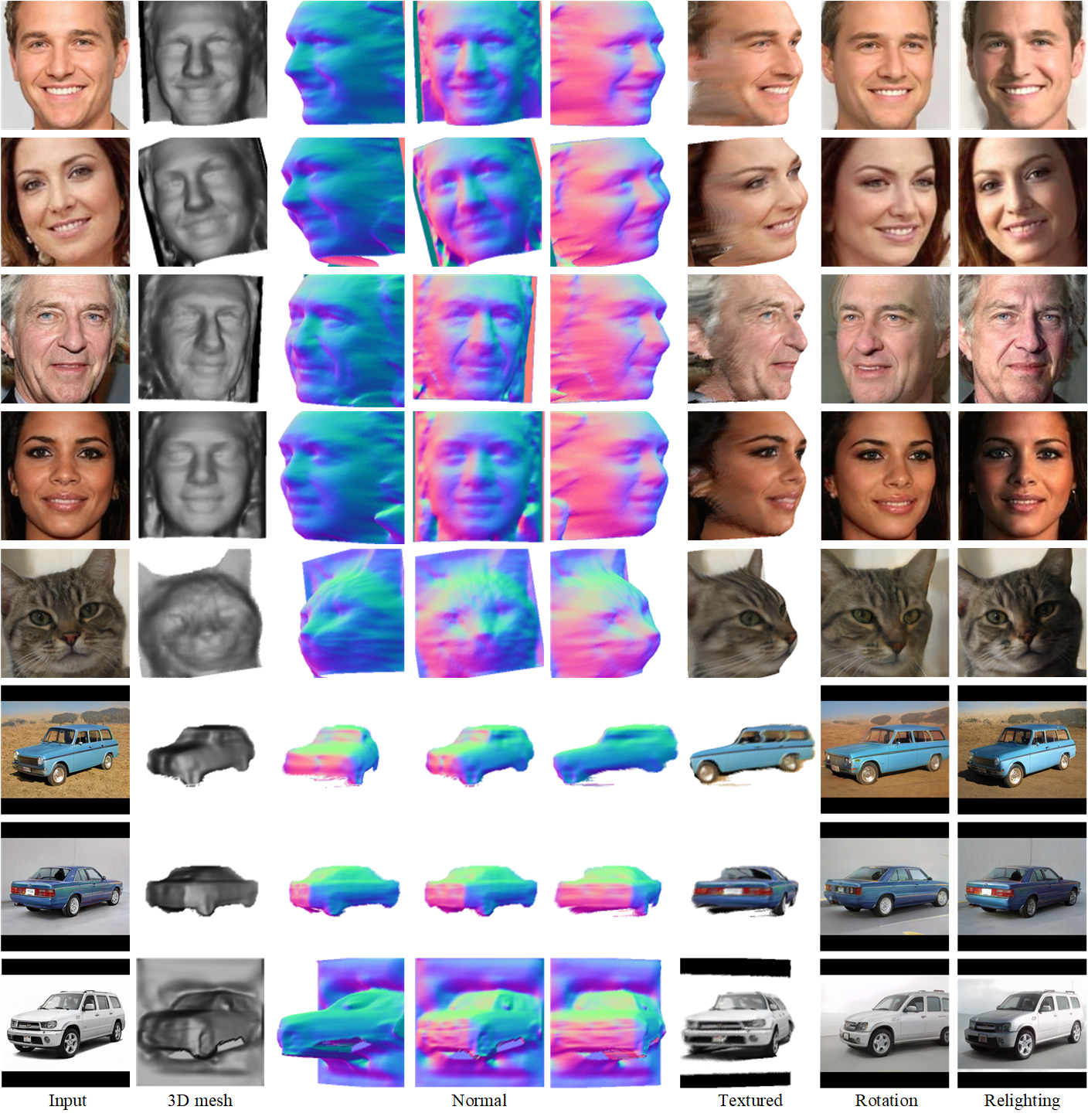
Materials
Video
Code
Citation
@inproceedings{pan2020gan2shape, title = {Do 2D GANs Know 3D Shape? Unsupervised 3D Shape Reconstruction from 2D Image GANs}, author = {Pan, Xingang and Dai, Bo and Liu, Ziwei and Loy, Chen Change and Luo, Ping}, booktitle = {ICLR}, year = {2021} }